Centralized Warehouse to synergize sporadic data sources for efficient emergency response
- Janak Parajuli, Suman Baral, Suresh Manandhar
- Nov 24, 2018
- 13 min read
Updated: Dec 4, 2023
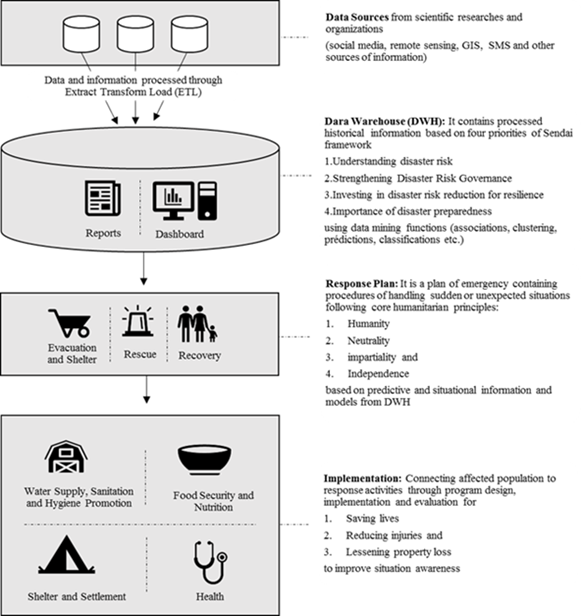
ABSTRACT
Natural Disaster is a natural phenomenon that may occur anytime or anywhere causing destruction of lives and properties further demanding the needs for immediate response. A timely effort is obligatory regarding the rescue and relief operations including resource management. Information and data from various sources begin to fly in the sky whose harmonized storage comes to be indispensable. Judicious extraction of information figuring out the patterns from the crude datasets ought to be done to facilitate sensible decision making by the process called data mining.
This paper is intended at conferring data warehouse as an effective means of emergency response. Data in a warehouse is acquired from diverse sources like social media (facebook and twitter hashtags, mostly), android applications, crowdsourcing, government bodies, relief providing organizations and so on. The collected data are then stockpiled in a central data warehouse using different schemes for database management system. Besides, data mining is also facilitated by data warehousing. These lumped data are analyzed using various approaches of pattern recognition and predictive modelling. In general, primarily scrutinized data involves finding the attribute and spatial figures of injured, casualties and missing ones. In addition, other analyses are done to figure out the suitable location for IDP camps, food and relief materials. The data then can be used by the stakeholders (government agencies, NGOs, INGOs and so on) as per their necessities.
To conclude, here we can perceive that emergency response and management is a synchronized approach involving different stakeholders. A proficient mining of centralized warehouse datasets acquired from various sources can be a milestone for the speedy disaster management.
Key words: Emergency, Warehouse, social media, pattern recognition, data mining, IDP camps
Introduction
Natural disaster is the phenomenon that may occur anytime or anywhere causing destruction of lives and properties while demanding the needs for immediate response. According to Ministry of Home Affairs, more than 12000 people have lost their life due to natural disasters in the last seven years in Nepal[4]. Understanding and tracing the advancement of disaster and crisis situations are extremely difficult due to their complexity. Disaster management is not only related to prediction of consequences of disasters but also principally directed at minimalizing the undesired results from disasters in short time. Nowadays, modern technologies have evolved to support people to make apt decisions to confront disaster scenario. So disaster management is no exception, however, it has its own challenges and defies because of the unprecedented volume of data (A.T. Zagorecki et al., 2013, pp. 353). The disaster management or the emergency management is the process which assimilates different available resources to administer emergency analyzing its root, growth and results (Ji, L. and Chi, H. 2006).
In order to make timely rescue operations and proper resource management, systematic analysis of acquired information must be done which can be achieved by the process called data mining (Goswami et al., 2018 pp. 367). Discovering interesting and useful patterns from large datasets and extracting the hidden information from them using set of rules is data mining. Other terms used for data mining are knowledge discovery (mining) in databases (KDD), knowledge extraction, data/pattern analysis, data archeology, data dredging and information harvesting (Jiawei, H. et al, 2011)(Fayyad, U. et. al.; 1996)(Tan, P. et. al., 2005). This data mining process is carried out to build an effectual predictive or descriptive model of a large amount of data that not only best fits or explains it, but is also able to generalize to new data (Mukhopadhyay, A., 2014, pp. 6). Additionally, in data mining we discover interesting knowledge from large amounts of data stored in either databases, data warehouses, or other information repositories through some kind of algorithms (Chen, F. et. al., 2015).
Background
This research is oriented towards conferring centralized warehouse and data mining techniques as efficient approach of emergency response. Warehouse accumulates the data from various sources like social media (facebook, twitter), remote sensing imageries, crowdsourcing, android application, relief organizations (like Red Cross) etc. In today’s scenario, mining planned and anticipated data from social media feeds have become more successful in providing valuable intuitions and situational awareness to the first responders of the disaster (Vieweg, S., 2012. These data are then scrutinized using various approaches of pattern recognition and predictive modelling with preference to four priorities and seven targets of Sendai Framework. Based on data mining techniques for predictive and situational models, we can plan for rescue, recovery, evacuation camps, food and shelter. There are several major data mining techniques developed and used: association, classification, clustering, prediction, sequential patterns and decision tree. We often combine two or more of those data mining techniques together to form an appropriate process that meets the humanitarian needs[5]. The analyzed data then can be disseminated with preference to the four primary Sphere indicators.
ICTs in Disaster Response
Parajuli, J. and Baral, S., 2018 in page number 2-4 have talked about wide scope mobilization of ICTs in disaster (flood, landslide, forest fire, earthquake etc.) response and management throughout the world. The main advantage of ICTs is they play prominent role in difficult situations where human involvement for rescue purpose is perilous. It is obvious that ICTs can’t replace the need for food, water, shelter etc. in times of disaster but we can’t deny the fact that it has become the middleware for worldwide collaboration[6]. ICTs help to reach large number of victims in lesser time more efficiently and help the communities to be more pliable and prepared for the anticipated disasters in future. Besides, smartphones and mobile applications have risen to be the efficacious and fastest means of communication during disaster period. They have defied all the hurdles to empower incessant connectivity during the times when we need them most providing a new effectual way of communication in order to save more lives.
A Standard Approach to Emergency Response
The main focus of this research is to recommend the Consolidated Data Warehouse as the efficient emergency response scheme. Data collection is done from varying sources including Remote Sensing imageries, UAVs, Mobile SMS and android applications etc. to develop a consolidated data warehouse. At present, there is this growing trend of mining social media data also, for response and relief purpose from the research proficient (Imran, M. et. al., 2013). The rudimentary idea is to cluster these data in a warehouse, analyze them and distribute to the stakeholders including government and humanitarian organizations to save lives, livestock and properties.
Data Warehouse characteristics
Unlike database system, data warehouse is only for storage, query and analysis of the data stored from single or multiple sources (including databases also) in a cyclic manner and not frequently. Databases are concurrent storehouses of information and usually they are collected for particular purposes. According to Business Management Ideas, a warehouse generally functions in three steps. In the first step, raw data are stored for use by developers called as staging. The next is data integration step in which obtained data are assimilated to build up a general concept by users. In the third step, data are then cleaned, transformed and categorized and made accessible to the users in this last step called access[7]. Commonly the characteristics of data warehouse can be listed in following four points:
i. Subject-oriented: Data in a warehouse are obtained from various sources and they should be cleaned and transformed into a uniform format abiding by naming conventions, formats and encoding.
ii. Integrated: In a warehouse, data from several sources are integrated.
iii. Non-volatile: The data are for read only purpose without supporting CRUD operations and even old data are not erased while adding newer which helps to find out the historical trends of data.
iv. Time variant: Principally, the data in a warehouse are kept concerning the historical point of view which helps to study trends and changes.
When we talk about disaster risk reduction and response, basically we need to emphasize on two popularly accepted approaches by United Nations: Sendai framework and Sphere standards.
i. Sendai Framework: This is the foremost major covenant endorsed by UN General Assembly for the 15 year period during 2015-2030 aimed at disaster risk reduction[8]. It adopts four priorities and seven targets for substantial minimization of disaster menace. It emphasizes on understanding all the dimensions (vulnerability, exposure to hazards etc.) of the disaster risk, strengthening the governance in disaster risk and its management, encouraging investments in disaster risk reduction for pliability and finally enhancing disaster vigilance for efficacious response with 3B (Build Back Better) approach in recovery, rehabilitation and reconstruction (Twigg J., 2015, pp. 329).
ii. The Sphere Standard: According to Sphere Handbook-2018, the Sphere Standards were created by a Red Cross and Red Crescent movement in collaboration with a group of altruistic people working in non-governmental organizations. It also claims that the philosophy of Sphere resides on two main beliefs:
Every people who are affected by disaster have right to life and assistance with self-respect.
Necessary precautions and steps ought to be taken to lessen human suffering due to disaster.
Further, it has set minimum standards in four vital response areas which are Health, Food Security and Nutrition, Shelter and Settlement and lastly the Water Supply, Sanitation and Hygiene Promotion (WASH).
This research is concentrated on proposing the emergency response model within the scope of these two approaches accepted by United Nations and humanitarian organizations.
Proposed Disaster Response Model
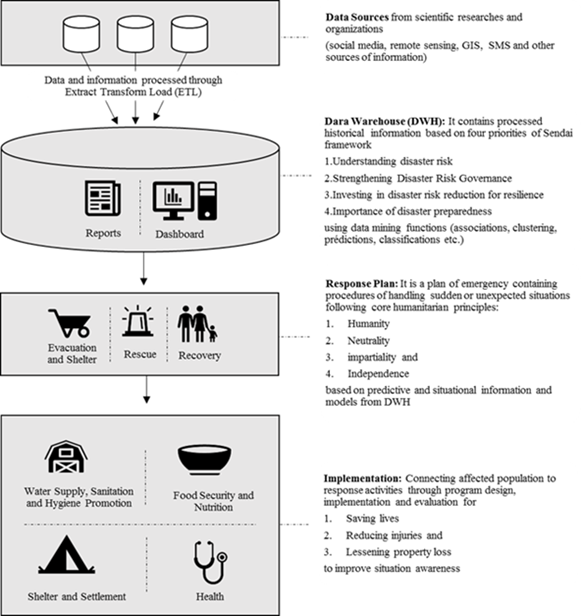
Figure 1. A proposed framework for efficient disaster response
[endif]--Our proposed model to efficiently respond emergency can be summarized in following four components: data sources, warehouse, response plan and implementation.
i. Data Sources: There are various contributors for data including scientific community, researchers, humanitarian organizations, social media (facebook, twitter etc.), remote sensing imageries, SMS and mobile applications etc. The question is how these data can be managed properly in order to utilize them for the betterment of mankind during disasters. Disaster time is crucial in the sense that response time is very less and in that time saving time effectively is saving lives. In this sense we can claim that time is inversely proportional to number of lives. According to world economic forum, during 2005-2014 more than 7,00,000 lost their lives by disaster and obviously it could have been minimized, had some precautions been taken[9]. So, basically there is this requisite of harmonized storage of those data. The next thing we need to be aware is those data from various data are inconsistent and varies in terms of format and so they can’t be used as such. To make it usable, we need to bring consistency in data formats and information products.
ii. Data Warehouse: So as to address aforesaid issues, we have come up with the notion of data warehouse which can play vital role not only in efficient disaster response but also in mitigating the disaster risk for future purposes. The collected data are then processed through a method called Extract Transform Load (ETL). The extract, transform and load are three database functions integrated into single means to excerpt data out from one database into another database. During extraction, the data are read from different sources and collected in a vessel. The data obtained from various sources are not uniform so the next step is to standardize those using predefined norms and rules or lookup tables. Lastly, the unified data are then loaded into the target database. All these process are done using different data mining functions like associations, clustering, predictions, classifications, modelling etc. The data can be visualized in dashboard in terms of bar graphs, charts, reports, tables and other statistical representations. Users can query the database dynamically and can access data as per their requirements, however, since the data are read only, they can’t run CRUD operations which is outside the notion of data warehouse.
iii. Response plan: Using the data from the warehouse, stakeholders including government, humanitarian organizations like Red Cross, Scout etc., media and general public can make either a timely escape plan or plan to tackle the disaster. Basically the rescue operations are carried out following the four humanitarian principles: humanity, neutrality, impartiality and independence[10][11]. Also, these data can be analyzed using various approaches of pattern recognition and predictive modelling to find out the figures of injured, casualties and missing ones. Similarly, the number of internally displaced people (IDP) can be determined to calculate the required count and apposite location of evacuation camps and shelter. Besides, it will be very easy to supply food, medicine and relief materials also. Not only this, the mobilization of warehouse data can be protracted for recovery and rehabilitation approaches as well.
iv. Implementation: In the implementation phase, affected people can be connected to response activities and encouraged them for saving lives, reducing injuries and minimizing the property loss to enhance situation awareness. Within the scope of sphere standards, programs can be launched in order to improvise the condition of: a. water supply, sanitation and hygiene promotion, b. food security and nutrition, c. shelter and settlement and d. Health through coordination and collaboration between organizations.
Data and information in the warehouse
Data warehouse is the storage location of both historical and current data sources and processed information as insights. According to infogineering, although data and information seem to be used interchangeably, their major difference is that data is raw material that is to be processed to acquire anticipated information i.e. data is used as input for the model, algorithms and processes while information is the output of data[12]. As been discussed already, the data comes from various sectoral data sources as remote sensing and UAV, social media, GIS databases, research and academia reports, multimedia, micro-mapping etc. These technologies often reach and inform about the situation where communication, location and rescue is risky or even impossible. To give a clear sense of how these all fit into disaster response, here we have considered a use case for flood which is one of the supreme taking place disaster and most often pertinent in most part of world.
The list of anticipated data and information is as follow:-
Data: It is the input for the model, fitted as values for variables. In this case we may need the following but not limiting to these only, data from various sources clustered in a warehouse:
Hydrological and meteorological records
Population and infrastructure
Vulnerabilities, capacities and exposed hazards
Local clubs, Organizations, Community based Organizations (CBO) including first responders as set by government and non-government organizations
Education institutions, government and non-government organizations disaggregated by working sector and area
Early warning data containing flood level, location of flood
Social media reporting standards - hashtags, safety checks, tags etc.
Real time cellular locations
Sewage and drainage channel
Network of micro-mappers
Information and process: Most of the information during the process is automatic rather than manual i.e. aforesaid data are processed using various algorithms and models to acquire desired information. The said data can be processed to get following, and again not limiting to these only, information:
Weather forecast as per hydrological and meteorological records from stations
Early warning dissemination channels to reach people to be affected with time limit of flood to reach from one early warning station to another that disseminated the level and extent of flood
Communication of safety measures through radio, television and faster popular means. This may also include notification of individuals through social media.
Appropriate location identification for internally displaced people (IDP) using GPS, GIS and remote sensing technology
Deploying first responders in the field based on information from micro-mappers including response channel, evacuation routes and its classification
Mathematical model for loss estimation. The loss primarilyy includes human, property and livestock loss calculated based on previously available data in the warehouse.
Dissemination of disaggregated data developed based on previously fetched information that indicates organization roles, working area, sector and indicator as per Sphere standards.
Advantages/limitations of Warehouse
It is an intricate job to examine and evaluate the efficaciousness of a disaster response system in a quantitative manner since at present there are no any protocols to quantity it (Chapman, C., and Ciravegna, F., pp.7). However we can weigh the system in terms of its pros over cons. While talking about its pros, data warehouses do have uniform data quality with quicker and easier data access and thus assist in better decision making name="_ftnref13" title=""[13]. Other pros are, data history are maintained to study chronological trends, and the data integrity is maintained with standard norms, codes and rules. Thus, the large volume of data are cleaned by removing inconsistent errors and improves the data quality of metadata. Indexing is done to deal with large volumes of data so as to increase data accessing speed. Irrespective of the data sources, there is a common data model for all sorts of data. Data are reorganized as per requirements making easy for complex analytic queries and during all these also, operational systems are intact. Additionally, unauthorized access are checked with data security policies and users can only access data through various pre-defined modules[14].
As it is palpable that, every coin has two sides. Though, there are many advantages of data warehousing, there are some drawbacks as well. Data are obtained from various sources due to which the major drawback regarding the ownership, privacy and secured results occurs at this point. Besides, all those data may not be compatible each time and the technicians may have to go through the specific cases of data resulting in consumption a significant amount of time. Normally, an organization runs warehouse data at the risk of mapping in with same schema and filters which diminishes the pliability of data. It is difficult job to maintain such a tremendous volume of data online.
Conclusion
In summary, here we discussed the potential and challenges of data warehouse as an efficient emergency response schema and this paper is oriented towards conferring it as an efficient approach. Data warehouse is the suitable answer to handling large volume of data as it cleans and standardizes them to aid responders form a quick action plan at the time of emergency. The data are analyzed within the scope of four priorities and seven targets of Sendai framework, a commonly endorsed covenant by the UN using pattern recognition and predictive modelling techniques. In addition, using these techniques we can derive apt plan for timely response for rescue and relief operations along with “Build Back Better” approach in recovery, rehabilitation and reconstruction. The derived information acquired after processing the available data in the warehouse, can be disseminated sector wise so that the emergency responders can use it as per their requirement. While responding, normally they endure within the scope of standards set by Sphere Standards, another commonly endorsed covenant by the UN. Establishing the two main beliefs regarding people’s right to life and precautions to be taken to lessen human suffering during disaster, the sphere standard has also set protocols in four vital areas which are Health, Food Security and Nutrition, Shelter and Settlement and lastly the Water Supply, Sanitation and Hygiene Promotion (WASH).
References
Chapman, S. and Ciravegna, F., “Focused Data Mining for decision support in Emergency Response Scenarios” funded by Advanced Knowledge Technologies (AKT) Interdisciplinary Research Collaboration (IRC) sponsored by EPSRC, grant number GR/N15764/01, pp. 1-9
Chen, F.; Deng, P.; Wan, J.; Zhang, D.; Vasilakos,A.V.; and Rong, X.; (2015) ‘Data Mining for the Internet of Things: Literature Review and Challenges’, Hindawi Publishing Corporation International Journal of Distributed Sensor Networks Volume 2015, Article ID 431047, 14 pages http://dx.doi.org/10.1155/2015/431047
Fayyad, U.; Piatetesky-Shaoiro, G.; and Smyth, P. (1996) “From data mining to knowledge discovery in databases,” AI Magazine Vol. 17, No.3, 1996.
Goswamia S., Chakrabortya S., Ghosha S., Chakrabarti A., Chakraborty B., (2018) ‘A review on application of data mining techniques to combat natural disasters’, Ain Shams Engineering Journal, ISSN: 2090-4479, Vol: 9, Issue: 3, Page: 365-378
Imran, M.; Elbassuoni, S.; Castillo, C.; Diaz, F.; Meier, P. (2013) “Practical extraction of disaster-relevant information from social media”, In Proceedings of the 22nd International Conference on World Wide Web Companion, Rio de Janeiro, Brazil.
Ji, L. and Chi, H. (2006). “Emergency Management”, High Education Press. Jiawei, H.; Kamber, M. and Pei, J. (2011) ‘Data Mining: Concepts and Techniques 2011’ Morgan Kaufmann Publishers Third edition.
Mukhopadhyay A., Maulik U., Bandyopadhyay S. and Coello C. A. C, (2014) "A Survey of Multiobjective Evolutionary Algorithms for Data Mining: Part I", in IEEE Transactions on Evolutionary Computation, vol. 18, no. 1, pp. 4-19, doi: 10.1109/TEVC.2013.2290086
Parajuli, J.; Baral, S. (2018) “Remote Sensing Schemes Mingled with Information and Communication Technologies (ICTS) for Flood Disaster Management”, i-manager's Journal on Pattern Recognition; Nagercoil Vol. 4, Iss. 4, (Dec 2017-Feb 2018): 1-9.DOI:10.26634/jpr.4.4.14128
Tan, P.-N., Steinbach, M., & Kumar, V. (2005)“Introduction to Data Mining”, Addison Wesley, Twigg, J. (2015), “Disaster Risk Reduction”, Overseas Development Institute, 203 Blackfriars Road London SE1 8NJ United Kingdom, new edition, pp.1-382
Vieweg, S. (2012) “Situational Awareness in Mass Emergency: A Behavioral and Linguistic Analysis of Microblogged Communications”. Ph.D. Thesis, University of Colorado at Boulder, Boulder, CO, USA.
Zagorecki, A.T., Johnson, D.E.A. and Ristvej, J. (2013) ‘Data mining and machine learning in the context of disaster and crisis management’, Int. J. Emergency Management, Vol. 9, No. 4, pp.351–365. /
URLs
[4]http://www.neoc.gov.np/uploads/news/file/Seven%20year%20data_20180427010733.pdf, retrieved on 25th Jan, 2018.
[5]http://www.zentut.com/data-mining/data-mining-techniques/ retrieved on 25th Jan, 2018
[6]https://www.forbes.com/sites/trevornace/2017/12/15/how-technology-is-advancing-emergency-response-and-survival-during-natural-disasters/#2560d3dd9cc8 retrieved on 15th Dec, 2017, 01:31 pm
[7]http://www.businessmanagementideas.com/crm/customer-database/data-warehouse-meaning-characteristics-and-benefits/3608 retrieved on 25th Dec, 2017
[8]https://www.unisdr.org/we/coordinate/sendai-framework, retrieved on 20th Dec, 2017
[9] https://www.weforum.org/agenda/2018/01/4-ways-technology-can-play-a-critical-role-in-disaster-response/ retrieved on 26th Jan, 2018
[10] (The Sphere Handbook- 2018)
[11]https://www.unocha.org/sites/dms/Documents/OOM-humanitarianprinciples_eng_June12.pdf retrieved on 27th Jan, 2018
[12] http://www.infogineering.net/data-information-knowledge.htm retrieved on 26th Jan, 2018
[13] https://dzone.com/articles/data-warehouse-characteristics-and-benefits retrieved on 25th Dec, 2017
[14] https://content.wisestep.com/data-warehousing-characteristics-functions-pros-cons/ retrieved on 25th Dec, 2017
![endif]--
Comments